Recursive Deep Models for Semantic Compositionality Over a Sentiment Treebank
An easy tutorial about Sentiment Analysis with Deep Learning and Keras by Sergio Virahonda
However, while a computer can answer and respond to simple questions, recent innovations also let them learn and understand human emotions. So, after that, the obtained vectors are just multiplied to obtain 1 result. Every weight matrix with h has dimension (64 x 64) and Every weight matrix with x has dimension (100 x 64).
Using sentiment analysis, businesses can study the reaction of a target audience to their competitors’ marketing campaigns and implement the same strategy. Financial firms can divide consumer sentiment data to examine customers’ opinions about their experiences with a bank along with services and products. To put it in another way – text analytics is about “on the face of it”, while sentiment analysis goes beyond, and gets into the emotional terrain. What keeps happening in enterprises is the constant inflow of vast amounts of unstructured data generated from various channels – from talking to customers or leads to social media reactions, and so on. Sentiment analysis is a vast topic, and it can be intimidating to get started. Luckily, there are many useful resources, from helpful tutorials to all kinds of free online tools, to help you take your first steps.
GPT VS Traditional NLP in Financial Sentiment Analysis – DataDrivenInvestor
GPT VS Traditional NLP in Financial Sentiment Analysis.
Posted: Mon, 19 Feb 2024 08:00:00 GMT [source]
‘ngram_range’ is a parameter, which we use to give importance to the combination of words, such as, “social media” has a different meaning than “social” and “media” separately. Change the different forms of a word into a single item called a lemma. Stopwords are commonly used words in a sentence such as “the”, “an”, “to” etc. which do not add much value. Because, without converting to lowercase, it will cause an issue when we will create vectors of these words, as two different vectors will be created for the same word which we don’t want to.
This resulted in a significant decrease in negative reviews and an increase in average star ratings. Additionally, Duolingo’s proactive approach to customer service improved brand image and user satisfaction. The sentiments happy, sad, angry, upset, jolly, pleasant, and so on come under emotion detection. In the case of movie_reviews, each file corresponds to a single review.
Step 6 — Preparing Data for the Model
Businesses use these scores to identify customers as promoters, passives, or detractors. The goal is to identify overall customer experience, and find ways to elevate all customers to “promoter” level, where they, theoretically, will buy more, stay longer, and refer other customers. Brand monitoring offers a wealth of insights from conversations happening about your brand from all over the internet.
Market research is a valuable tool for understanding your customers, competitors, and industry trends. But how do you make sense of the vast amount of text data that market research generates, such as surveys, reviews, social media posts, and reports? Natural language processing (NLP) is a branch of data analysis and machine learning that can help you extract meaningful information from unstructured text data. In this article, you will learn how to use NLP to perform some common tasks in market research, such as sentiment analysis, topic modeling, and text summarization. LSTMs and other recurrent neural networksRNNs are probably the most commonly used deep learning models for NLP and with good reason. Because these networks are recurrent, they are ideal for working with sequential data such as text.
Free Online Sentiment Analysis Tools
So, there may be some words in the test samples which are not present in the vocabulary, they are ignored. As we can see that our model performed very well in classifying the sentiments, with an Accuracy score, Precision and Recall of approx. And the roc curve and confusion matrix are great as well which means that our model can classify the labels accurately, with fewer chances of error. LSTM network is fed by input data from the current time instance and output of hidden layer from the previous time instance.
This analysis can reveal customer sentiments, trends, and patterns that inform decision-making, improve customer service, enhance product development, and drive marketing strategies. It’s a powerful tool for gaining a competitive edge and understanding market dynamics. Odin Answers is an AI-powered document analysis platform that uses machine learning and advanced statistics to find relationships and patterns in structured and unstructured data. The tool can effectively track and identify emotion and sentiment, including psychological attributes like trust, anger, and fear. Glean is one of the best AI tools for quickly and accurately locating information on any document or website. The analytics tool uses deep learning-based LLMs to understand natural language queries and constantly learns from your company’s unique language and context to provide more relevant results.
Each annotator has input(s) annotation(s) and outputs new annotation. Spark NLP comes with 20,000+ pretrained pipelines and models in more than 250+ languages. It supports most of the NLP tasks and provides modules that can be used seamlessly in a cluster. It is built on top of Apache Spark and Spark ML and provides simple, performant & accurate NLP annotations for machine learning pipelines that can scale easily in a distributed environment. Now that you’ve tested both positive and negative sentiments, update the variable to test a more complex sentiment like sarcasm.
Add the following code to convert the tweets from a list of cleaned tokens to dictionaries with keys as the tokens and True as values. The corresponding dictionaries are stored in positive_tokens_for_model and negative_tokens_for_model. Noise is specific to each project, so what constitutes noise in one project may not be in a different project. For instance, the most common words in a language are called stop words.
I’m sure that if you dedicate yourself to adjust them then will get a very good result. In the code above, we define that the max_features should be 2500, which means that it only uses the 2500 most frequently occurring words to create a “bag of words” feature vector. Words that occur less frequently are not very useful for classification. Enough of the exploratory data analysis, our next step is to perform some preprocessing on the data and then convert the numeric data into text data as shown below. Two new columns of subjectivity and polarity are added to the data frame.
The second approach is a bit easier and more straightforward, it uses AutoNLP, a tool to automatically train, evaluate and deploy state-of-the-art NLP models without code or ML experience. It’s notable for the fact that it contains over 11,000 sentences, which were extracted from movie reviews and accurately parsed into labeled parse trees. This allows recursive models to train on each level in the tree, allowing them to predict the sentiment first for sub-phrases in the sentence and then for the sentence as a whole. The first step in developing any model is gathering a suitable source of training data, and sentiment analysis is no exception. There are a few standard datasets in the field that are often used to benchmark models and compare accuracies, but new datasets are being developed every day as labeled data continues to become available.
Before proceeding to the next step, make sure you comment out the last line of the script that prints the top ten tokens. There are certain issues that might arise during the preprocessing of text. For instance, words without spaces (“iLoveYou”) will be treated as one and it can be difficult to separate such words.
To make statistical algorithms work with text, we first have to convert text to numbers. In this section, we will discuss the bag of words and TF-IDF scheme. If we look at our dataset, the 11th column contains the tweet text.
- DataRobot customers include 40% of the Fortune 50, 8 of top 10 US banks, 7 of the top 10 pharmaceutical companies, 7 of the top 10 telcos, 5 of top 10 global manufacturers.
- After performing this analysis, we can say what type of popularity this show got.
- In the next article I’ll be showing how to perform topic modeling with Scikit-Learn, which is an unsupervised technique to analyze large volumes of text data by clustering the documents into groups.
This makes it an invaluable tool for digital marketers and content creators who often have difficulty optimizing AI-generated content. CoCouncel works on the GPT-4 framework – the same model that outperformed real bar candidates shortly after launch. Sentiment Analysis in NLP, is used to determine the sentiment expressed in a piece of text, such as a review, comment, or social media post. Multilingual consists of different languages where the classification needs to be done as positive, negative, and neutral. Now you’ve reached over 73 percent accuracy before even adding a second feature!
All these classes have a number of utilities to give you information about all identified collocations. Note that .concordance() already ignores case, allowing you to see the context of all case variants of a word in order of appearance. Note also that this function doesn’t show you the location of each word in the text.
This is an extractor for the task, so we have the embeddings and the words in a line. So, we just compare the words to pick out the indices in our dataset. Take the vectors and place them in the embedding matrix at an index corresponding to the index of the word in our dataset. The number of nodes in the hidden layer is equal to the embedding dimension. So, say if there are 10k words in vocabulary and 300 nodes in the hidden layer, each node in the hidden layer will have an array of weights of the dimension of 10k for each word after training. Sentiment Analysis is a sub-field of NLP and together with the help of machine learning techniques, it tries to identify and extract the insights from the data.
From the output, you can see that our algorithm achieved an accuracy of 75.30. The original web application for producing and sharing computational documents is Jupyter Notebook. It provides a straightforward, simplified, and document-focused environment. This analysis gives them a clear idea of which regions need improvement. Now, there’s the need for machines, too, to understand them to find patterns in the data and give feedback to the analysts.
Sentiment Analysis: First Steps With Python’s NLTK Library
In the next step you will analyze the data to find the most common words in your sample dataset. In this tutorial you will use the process of lemmatization, which normalizes a word with the context of vocabulary and morphological analysis of words in text. The lemmatization algorithm analyzes the structure of the word and its context to convert it to a normalized form. A comparison of stemming and lemmatization ultimately comes down to a trade off between speed and accuracy.
Sentiment analysis is a popular natural language processing (NLP) task that involves determining the sentiment of a given text, whether it is positive, negative, or neutral. With the rise of social media platforms and online reviews, sentiment analysis has become increasingly important for businesses to understand their customers’ opinions and make informed decisions. However, there are still some challenges in sentiment analysis that deep learning models need to address. These include handling imbalanced datasets, dealing with sarcasm, irony, and figurative language, and incorporating domain-specific knowledge.
These two data passes through various activation functions and valves in the network before reaching the output. The sentiment analysis is one of the most commonly performed NLP tasks as it helps determine overall public opinion about a certain topic. It is evident from the output that for almost all the airlines, the majority of the tweets are negative, followed by neutral and positive tweets. Virgin America is probably the only airline where the ratio of the three sentiments is somewhat similar. Given tweets about six US airlines, the task is to predict whether a tweet contains positive, negative, or neutral sentiment about the airline.
Test Data Transformation
In this article, we will discuss using a pretrained Deep Learning (DL) model and then training a model, which chains together algorithms that aim to simulate how the human brain works. Noise is any part of the text that does not add meaning or information to data. Data security is a critical concern for AI text analysis tools, and reputable providers implement stringent security measures to protect the data. This includes encryption, secure data storage, and compliance with privacy regulations such as GDPR. It’s important to review the security policies of any AI text analysis tool before implementation to ensure it meets your organization’s security standards. Besides functioning as an AI writing assistant, PopAI has an on-page optimization tool designed to revolutionize the SEO production process and streamline your content production workflow.
Hence, we are converting all occurrences of the same lexeme to their respective lemma. As the name suggests, it means to identify the view or emotion behind a situation. Semantic Scholar is a free, AI-powered research tool for scientific literature, based at the Allen Institute for AI. This step involves looking out for the meaning of words from the dictionary and checking whether the words are meaningful. In NLP, such statistical methods can be applied to solve problems such as spam detection or finding bugs in software code. Sequences that are shorter than num_timesteps are padded with value until they are num_timesteps long.
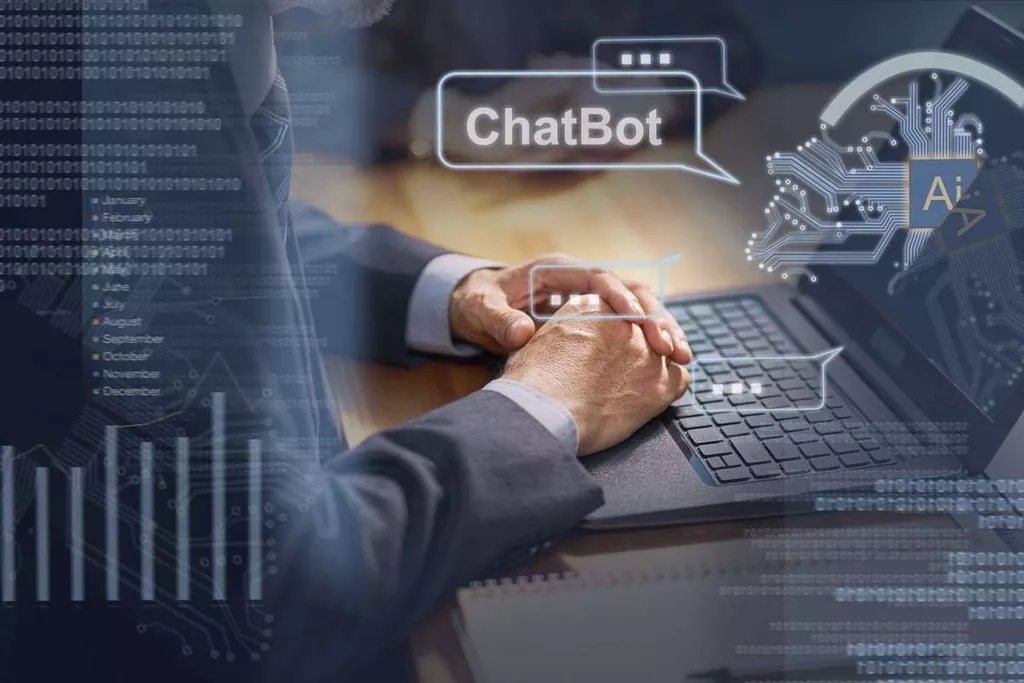
To be honest, RMSprop or Adam should be enough in most of the cases. As loss function, I use categorical_crossentropy (Check the table) that is typically used when you’re dealing with multiclass classification tasks. In the other hand, you would use binary_crossentropy when binary classification is required. In the next article I’ll be showing how to perform topic modeling with Scikit-Learn, which is an unsupervised technique to analyze large volumes of text data by clustering the documents into groups. This is defined as splitting the tweets based on the polarity score into positive, neutral, or negative.
In this article, we will focus on the sentiment analysis of text data. RNNs can also be greatly improved by the incorporation of an attention mechanism, which is a separately trained component of the model. Attention helps a model to determine on which tokens in a sequence of text to apply its focus, thus allowing the model to consolidate more information over more timesteps. If you check the John Snow Lab Model’s Hub, you will see that there are more than 200 models about sentiment analysis. Various models can be used for sentiment analysis, but there are some key differences between them. Each step contains an annotator that performs a specific task such as tokenization, normalization, and dependency parsing.
Emotion detection sentiment analysis allows you to go beyond polarity to detect emotions, like happiness, frustration, anger, and sadness. For training, you will be using the Trainer API, which is optimized for fine-tuning Transformers🤗 models such as DistilBERT, BERT and RoBERTa. As a technique, sentiment analysis is both interesting and useful. Now, we will check for custom input as well and let our nlp for sentiment analysis model identify the sentiment of the input statement. We will evaluate our model using various metrics such as Accuracy Score, Precision Score, Recall Score, Confusion Matrix and create a roc curve to visualize how our model performed. We will pass this as a parameter to GridSearchCV to train our random forest classifier model using all possible combinations of these parameters to find the best model.
You give the algorithm a bunch of texts and then “teach” it to understand what certain words mean based on how people use those words together. According to equation 4, the output gate which decides the next hidden layer. The new c or cell state is formed by removing the unwanted information from the last step + accomplishments of the current time step. The tanh is here to squeeze the value between 1 to -1 to deal with the exploding and vanishing gradient.
The approach is that counts the number of positive and negative words in the given dataset. If the number of positive words is greater than the number of negative words then the sentiment is positive else vice-versa. The .train() and .accuracy() methods should receive different portions of the same list of features.
Most people would say that sentiment is positive for the first one and neutral for the second one, right? All predicates (adjectives, verbs, and some nouns) should not be treated the same with respect to how they create sentiment. Read on for a step-by-step walkthrough of how sentiment analysis works. Finally, we can take a look at Sentiment by Topic to begin to illustrate how sentiment analysis can take us even further into our data.
We resolve this issue by using Inverse Document Frequency, which is high if the word is rare and low if the word is common across the corpus. It’s time to try another type of architecture which even it’s not the best for text classification, it’s well known by achieving fantastic results when processing text datasets. It’s a very good number even when it’s a very simple model and I wasn’t focused on hyperparameter tuning.
Find out what aspects of the product performed most negatively and use it to your advantage. We already looked at how we can use sentiment analysis in terms of the broader VoC, so now we’ll dial in on customer service teams. Discover how we analyzed the sentiment of thousands of Facebook reviews, and transformed them into actionable insights.
This article may not be entirely up-to-date or refer to products and offerings no longer in existence. Training logs show the constant increase in the accuracy of the model. One of, if not THE cleanest, well-thought-out tutorials I have seen!
[1][2] Each person spends an average of 151 minutes interacting with content from different brands and influencers on social media. [3] Social media users engage with content by liking, sharing, and commenting on various issues and posts during these interactions. The positive sentiment majority indicates that the campaign resonated well with the target audience. Nike can focus on amplifying positive aspects and addressing concerns raised in negative comments. Nike, a leading sportswear brand, launched a new line of running shoes with the goal of reaching a younger audience.
You’ll need to pay special attention to character-level, as well as word-level, when performing sentiment analysis on tweets. You can foun additiona information about ai customer service and artificial intelligence and NLP. Automatic methods, contrary to rule-based systems, don’t rely on manually crafted rules, but on machine learning techniques. A sentiment analysis task is usually modeled as a classification problem, whereby a classifier is fed a text and returns a category, e.g. positive, negative, or neutral.